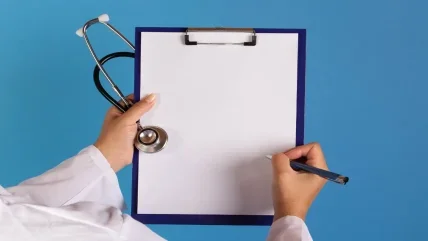
AI is all around us. We can wake up and ask smart speakers to tell us what the weather outside is like before we open the curtains. Then, if we need a bit of an energy boost, there are digital music services that can customise playlists based on our musical preferences in the time it takes us to press (or say) play.
If anyone truly appreciates the time and effort all that saves, it’s medical writers, who spend much of their days turning around clinical study reports (CSRs) as fast as humanly possible. A review of 78 of these mammoth documents published in the BMJ in 2013 recorded a mean length of 1,854 pages, but the most complex can stretch into the tens of thousands.
“Clinical trial reports are usually very long because they contain comprehensive critical information on safety, efficacy, methods and clinical results,” says Dr Nick Lynch of the Pistoia Alliance, a consortium of life science companies, including pharmaceutical manufacturers. “The level of detail required means
these reports take a long time for researchers and sponsors to complete before submission.”
According to surveys carried out by the European Medical Writers Association (EMWA) between 2008 and 2013, it takes an average of 16.9 working days (with a standard deviation of 8.2 and a range of 5–45) for a writing team to draft a CSR after receiving the final tables, figures and listings at the end of a moderately complex 200–400 subject phase-III study. That’s a quick turnaround, but in the race to get to market and start helping patients first, it could still be faster.
Sam Hamilton, former head of the EMWA, captured some of her peers’ frustrations memorably in the introduction to a 2008 piece called ‘Effective scheduling of clinical study reports’, in the EMWA journal The Write Stuff.
“For those of us working in a clinical-regulatory environment, there is a tacit understanding that even days shaved off a deliverable timeline can make ‘all the difference’,” she deadpanned. “But at whose expense? As a CSR author burning the candle at both ends, you probably realise you are not alone. Does the empathy of medical writers the world over alleviate your feelings of stress? Not even marginally?”
Where empathy fails, there’s always technology. One of the clearest use cases for AI in the latter stages of trials is in automating some of the most laborious aspects of producing first drafts of CSRs. Many of its pages require nothing more expert than identifying, collating and changing the tense of previous study documents, which doesn’t come close to utilising the scientific knowledge and talent of the medical writing team, but takes up a huge amount of their time.
However, by combining AI and content reuse technology, it could be possible to automate the production of 80% of the CSR body’s first draft, eventually cutting those 16.9 days down to as little as one or two, allowing medical writers to focus on clinical interpretation and complete the higher-value activities that require their unique knowledge and expertise.
Caffeine processors
“AI/ML [artificial intelligence/machine learning] has the potential to automate the creation of these reports and accelerate this process considerably,” explains Lynch, who also founded Curlew Research, which focuses on informatics, AI and digital strategy for health and life sciences, in 2014. “Algorithms that can ‘read’ trial results and data can automatically populate reports that a human reviewer can then check – requiring far less of their time. Given that clinical trials can typically take six to seven years to complete from phases I to IV, any technology that can shorten this time with the same or improved quality is welcome.”
Presently, completed CSRs are largely sponsorspecific marvels of coffee, teamwork and structured authoring. The last of those is a way of organising and identifying every element of a document according to a predefined, platform-independent system of tags. Those tags make it easier to delegate across a group of writers without overlooking anything, as well as making reading, understanding and using the information it contains as intuitive and efficient as possible.
As effective as structured authoring has proved in coordinating writing teams and managing their workloads, the format and core content of CSRs still differs substantially between sponsors, making reviews difficult for regulators, creating huge headaches during mergers and acquisitions, and frustrating efforts to increase transparency. That’s not too surprising given that the structures are primarily based on interpretations of the ICH E3 guideline, originally written in 1995, when internet connections still made robot noises on the phone line.
Nevertheless, as AI has advanced into our lives, collaborations like TransCelerate Biopharma’s Common CSR Template, and the medical writerdriven Clarity and Openness in Reporting: E3 (CORE)-based initiative that informed it, have started to standardise different sponsors’ approaches to presenting and organising information in CSRs. In doing so, they’ve laid the groundwork for a much greater degree of automation, which should, in turn, increase levels of standardisation. But there’s one hurdle left to leap.
“Currently, the majority of AI/ML, including natural language processing systems, are unable to recognise the context of data,” explains Lynch. No matter how powerful and well designed these ‘generic’ AI technologies might be, they are shaped by statistical analyses of public data sources, and thus have a limited facility for situating information in more bespoke, business or process-specific structures. So, while such systems might be able to identify an informed consent form or a statistical analysis plan, they would struggle to compute either’s relevance to a particular trial outcome.
The Pistoia Alliance specifically aims to test out emerging technologies like AI, so Lynch is well versed in what needs to happen for it to have a positive impact on clinical trials. As he puts it, “Automated contextual drafting aims to change this by training AI systems to recognise and take into account context when producing reports and outcomes.”
In this way, AI programs should be able to identify relevant information – whether it’s raw text, figures or tables – from previous study documents, and see how they relate and connect as outputs from trial planning, execution and results phases. From there, it would be relatively simple for these systems to transfer information to the right places in the draft CSR and make them retrospective.
“In the trials space, automation through AI/ML has already been explored in areas such as selecting eligible trial participants or monitoring compliance,” says Lynch. “Contextual drafting is the next step in accelerating the whole process. There is great promise, but it’s early days for AI/ML in clinical trials, and the idea of completely eliminating human researchers and scientists is some way off.”
Not the answer to everything
Indeed, for all that labour-saving potential, Lynch is quick to highlight what the technology can’t do. “Firstly, the garbage in-garbage out principle applies strongly, as it does in any scenario involving AI/ML,” he says. “Data quality is key to true outcomes – AI relies on harmonised and accurate data feeds to be successful.” Contextual AI can’t make up for laxity in any other trial aspects, or alchemise poorly recorded data into a winning submission at the last minute. “Secondly,” stresses Lynch, “although we are talking about a shift towards greater automation without human input, algorithms are essentially ‘trained’ and ‘tweaked’ by their human ‘masters’. Human nuance and inference are critical to understanding outputs, and AI should not simply be a ‘black box’ that spits out answers.” It’s a two-way street: we need to develop and maintain an adequate contextual understanding of how our AIs work too.
Moreover, to use AI/ML effectively, the pharmaceutical industry needs to vigilantly account for microscale issues like automation bias – where humans favour decisions made by AIs despite evidence showing they might be incorrect – and the macroscale possibility that these technologies, instead of improving the quality of CSRs, actually work to reinforce the outmoded practices and understandings that generated the data on which they’re trained.
“One area of risk is the introduction of bias into algorithm building,” Lynch adds. “Plurality and variety of data going ‘in’ is key, and researchers must be aware of the limitations of the data they employ. For example, adult males dominate the clinical trial population, representing about two thirds, and 86% of participants are white – even though the number of countries submitting clinical trial data to the FDA has almost doubled.” It will be necessary to regularly retrain AI with updated data sets to even begin the process of addressing this discrepancy, and, with it, adjusting to a more diverse trial population.
It might not take quite as concentrated an effort as producing a CSR, but keeping AIs up to date also requires specialised labour. In fact, Lynch points out that “successful AI/ML implementation requires skills and expertise that many biotech/life science companies don’t yet have access to”.
As the work of CORE and TransCelerate has shown, fixing and updating established practice in tightly regulated spaces like clinical trials takes collaboration. While the shortfall in programming and data expertise within individual pharmaceutical companies persists, AI looks likely to make that clearer than ever.
It is a belief the Pistoia Alliance is founded on. Lynch helps lead its Centre of Excellence for AI in Life Sciences and Health. “There is no doubt that AI/ML holds promise in the field of clinical trials,” he explains, “but to tackle such challenges, the industry needs to work together as one. Increased collaboration will allow the industry to find areas where AI can flourish, and where it can augment researchers working on clinical trials, as well as in areas such as the ‘lab of the future’. Sharing knowledge and resources will help us ensure that AI/ML boosts innovation while remaining safe and effective.”
Both absolutely vital to the success of a trial and set apart from its safe conduct, draft CSRs are a uniquely suitable focus area for improving the industry’s AI capabilities. No sponsor is going to recklessly experiment on the document that regulators use to determine whether or not a treatment will reach the market, but neither are missteps going to endanger trial participants.
“The benefit comes from the possibility that AI/ML will rapidly accelerate drug discovery, and ultimately, get much-needed treatments to patients faster,” says Lynch. But he couples that hope with a warning, “In clinical trials, where the safety of participants is paramount, AI/ML can augment human researchers but not replace them – and nor should we want it to.” Being told what the weather is like outside is no substitute for opening the curtains.
80%
Percentage of clinical study report draft production work that could be automated with contextual AI and content reuse technology.
Certara
1,854
The mean report page length of 78 clinical study reports used for a BMJ review in 2013.
BMJ