Drug discovery is a long, challenging and often futile process for companies like AstraZeneca – it’s estimated two-thirds of all clinical trials to find new medicines ultimately fail.
But that hasn’t stopped the global pharmaceutical firm attempting to discover new drugs more reliably and quicker with the same machine learning model Netflix uses to recommend TV shows and movies to its subscribers.
Dr Eliseo Papa, AI engineering lead at AstraZeneca, believes internal knowledge graphs can be used to more effectively structure and link together large amounts of data.
Why AstraZeneca needs to ramp up drug discovery
The knowledge graph technology is then used to “recommend” which new drugs should be targeted for research and clinical trials by scientists using the relevant parts of this data.
Speaking at the 2019 Spark + AI Summit in Amsterdam, Dr Papa said the rate at which the US Food and Drug Administration (FDA) approves new drugs has been steadily decreasing ever since the Thalidomide scandal in the 1950s.
He added: “It’s a pretty bleak picture. Drug discovery costs a fortune, and we’re really bad at choosing targets that will end up working.

“It takes ten years to see if your hypothesis is right, and two-thirds of drugs usually fail to make it to the market in the end – either through lack of efficacy or simply because too much time has elapsed without any success.”
However, Dr Papa said AstraZeneca is “working actively to change this from within”, and believes Netflix’s recommendation model could hold the key.
“Thanks to Netflix, we now know how to tackle these types of problems,” he said.
“Sometimes, selecting the next best drug target can be compared to choosing the next best movie – just with much more serious implications if you get it wrong.”
What are internal knowledge graphs? How they could help AstraZeneca with drug discovery
In a white paper published by tech company Semantic Web Company, knowledge graphs are defined as models to provide a structure to large amounts of data.
As an additional virtual layer lying on top of the existing data, it is therefore able to create a common interface between these databases, linking them all together.
This interface allows experts in the field to bring together structured data – information written in code – and unstructured data, which accounts for everything else.
This is important because of the vast amount of biological data that is manually typed or handwritten – a common form of unstructured data.
Rather than using folders and spreadsheets to uniformly categorise data, however, a knowledge graph does this by organising data in the same way as the human brain – through context and relations.
The fluidity of this structure means knowledge graphs can use machine learning to grow organically whenever new data is introduced, creating new relations between databases and adding more context.
By connecting data in this way, they allows users to make informed decisions based on all the available information, and find connections they might not have found otherwise.
Internal knowledge graphs allow big companies like Google, Amazon and Microsoft to bring together and harness the vast amounts of data they have accumulated over time.
Virtual assistants like Siri and Alexa use knowledge graph technology to respond to commands intelligently, and learn about their users.
The info box seen on the right-hand side of Google’s search results, and personalised recommendations when shopping online, are also made possible by internal knowledge graphs.
However, Dr Papa says Netflix is his preferred example when it comes to explaining the model AstraZeneca uses to target new drugs.
Why is AstraZeneca using Netflix’s recommendation model to target new drugs?
In 2018, the FDA accepted 137 new drug approvals (NDAs) and biologicals licence applications (BLAs) – representing an 11% increase on the previous year, according to research group GlobalData.
But Dr Papa believes methods of drug discovery can still be improved so that more prescription medicines get through clinical trials and ultimately make it to the commercial market.
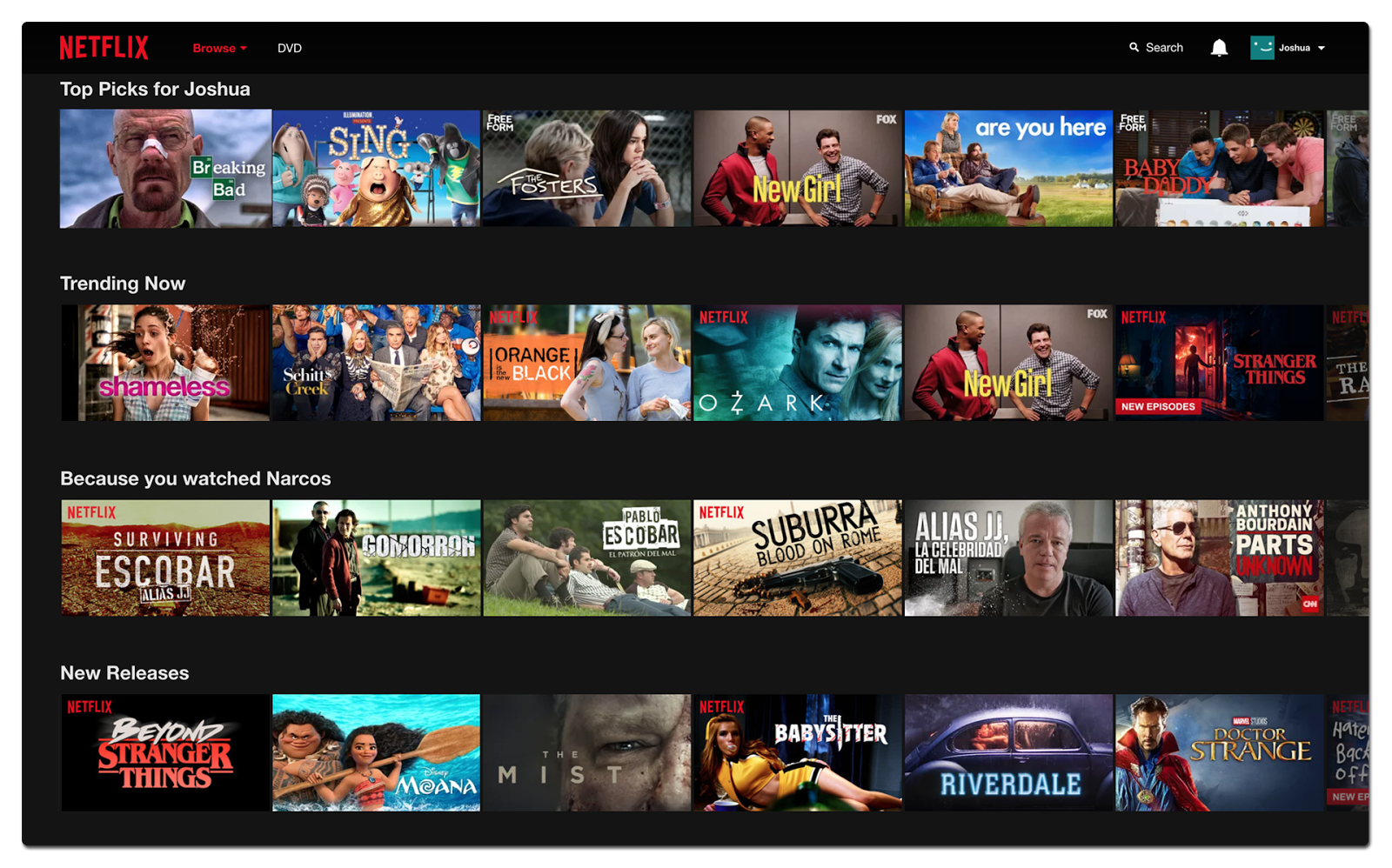
He said: “Currently, you give an idea to a scientist, they go into a lab, and maybe a year or two later they’ll let you know whether you were onto something.
“That feedback cycle is hard to break – but at AstraZeneca, we’re working on making this cycle shorter, and making better decisions.
“We’re basically trying to approach this by organising all the data, and then working on a recommendation system.”
Netflix’s recommendation system uses a similar model to direct its users towards content it believes they will enjoy based on a number of factors.
This recommendation system uses three main ways of filtering data in order to find a suitable target drug for scientists to begin testing – collaborative, content-based and knowledge-based.
Netflix | AstraZeneca | |
Collaborative filtering | Looks at what other people are watching online to recommend what its user should watch next | Uses information on how patients have responded to other medicines to select a target drug |
Content-based filtering | Looks at the user’s profile, their likes and dislikes etc, to decide which content it should recommend specifically for them | Looks at the characteristics of various drugs to decide which one should be targeted next |
Knowledge-based filtering | Traditionally used when the previous two approaches can’t be applied – looks at users, content and criteria to decide which content they would enjoy | Uses a more complex, detailed approach, looking at the recommendation criteria, the drugs and the patients, and the connections between them |
Once these filters have been applied, the resulting data can be used to find medical information and papers on a specific disease which would have otherwise been too difficult or too expensive to link together.
Dr Papa said scientists must embrace these uses of AI in the pharmaceutical industry because the amount of data they have to handle today is too large.
He said: “We need to be able to run this recommendation system at least every week to stay in sync with the rest of the scientific world.
“We think we’re sitting on a goldmine – 30-plus years of people exchanging thoughts on targeting new drugs.”
However, this relies upon bringing all the internal data AstraZeneca holds on pharmaceuticals together with the wealth of public data in the field – much of which is unstructured.
Dr Papa added: “There are a few start-ups and a lot of big companies that are now working on finding new target drugs using these systems.
“Many are trying to do this for rare diseases, on which they will often have no data.
“For example, some companies are trying to figure out what works in treating lung cancer, and then applying it to a rare form of ataxia, which has never been worked on before and only has 10 patients.
“That’s really important because pharmaceutical companies are happy to develop a drug just for 10 patients – as long as it isn’t too time-consuming or too expensive.”