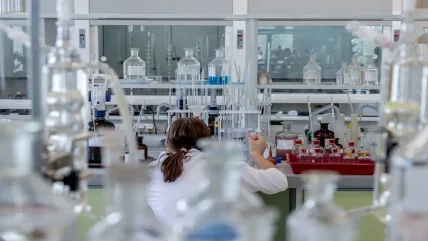
Whenever a clinical trial gets under way, it’s safe to say that all concerned are hoping for a successful result. However, with attrition rates high, approval is far from a given. According to a 2014 study, only 10% of all indications in phase I make it through to an FDA review, and the success rate for small-molecule drugs is lower still. In oncology, 93% of drug candidates fall before the final hurdle.
This being the case, it’s surely better to fail sooner rather than later. If a drug makes it all the way to phase III, it will have cost the sponsor company astonishing sums of money (sometimes as much as $1 billion), as well as many years of research and development. It will also have been trialled on numerous people, exposing them to all the associated liabilities.
This problem is only getting worse. Faced with higher regulatory hurdles for new drugs, and ever increasing trial cost and complexity, pharma companies continue to slam up against late-stage attrition. Of the drugs that do make it to phase III, 30-40% of them fail, followed by a not-negligible percentage at the NDA-to-approval stage.
The task, then, is to weed out poor candidates at the early stages, ensuring that time and money is diverted towards more promising avenues of research.
Proof of concept (POC) trials, covering phase I and IIa of clinical development, play a vital role in determining whether a treatment is likely to be effective, and whether it is worth pursuing further. Source the right information during this inexpensive stage of development, and you can spare a wasted investment further down the line.
How this can be accomplished, however, is a question in point. POC trials are designed not to confirm a drug’s efficacy but simply to provide early pointers and, as a result, they are necessarily narrow in scope. Because false positives can lead to late-stage attrition, and false negatives can mean dropping a genuinely beneficial drug, pharma companies must walk a fine line between two competing risks. They must somehow obtain a reasonable estimate of efficacy with relatively little information and few subjects.
A recent paper in PAIN explored possible ways round this problem, highlighting the most recent recommendations by the Initiative on Methods, Measurement, and Pain Assessment in Clinical Trials (IMMPACT). Focusing specifically on treatments for chronic pain, the IMMPACT group develops consensus reviews for improving clinical trials. The meeting covered here looked into POC trial designs, asking how their power might be maximised with limited resources and participants.
“The goal of the IMMPACT group is to improve methods for analgesic clinical trials,” explains Jennifer Gewandter, a research assistant professor at the University of Rochester and the lead study author. “The point of this meeting was to convene experts to discuss the different aspects of proof-of-concept clinical trial designs that should be considered, as well as the advantages and disadvantages of specific designs. Its ultimate goal was to disseminate the results of the consensus to pain researchers to improve the design of POC clinical trials in the field.”
Tough measures
Chronic pain is particularly difficult to study because it lacks the objective biomarkers of many other therapeutic fields. Because the primary outcome measure is a subjective rating of pain, it can be hard to work out which effects are due to the drug and which stem from more nebulous factors. Unsurprisingly, many analgesic drugs fail in phase III.
“We see high levels of inter-participant variability, which can make it difficult to see a difference between treatment groups,” says Gewandter. “Additionally, there is a large placebo effect in many chronic pain trials that is not as much of an issue when looking at things like blood pressure or cholesterol. Finally, many pain treatments work only for a subset of patients with the same condition. We are still not able to predict which patients will benefit most from each treatment, so it is sometimes difficult to see a difference between two groups even when a subset of participants may have truly benefitted.”
These trials typically adopt one of two design strategies – parallel or crossover designs. In a crossover trial, the same subjects receive a sequence of different treatments or exposures over time, whereas in a parallel trial, different treatments are administered to different groups.
Both have their advantages, but neither comes out as a clear-cut winner. In a crossover design, you stand to eliminate the problems associated with inter-patient variability – a pertinent factor in a pain trial. As a result, you can obtain a clearer idea about the treatments’ respective merits while recruiting fewer subjects. On the other hand, the accuracy may be open to question, owing to so-called ‘carryover effects’ where the treatment in the first period affects the results in the second.
“Carryover effects can decrease the ability to see a difference between groups,” explains Gewandter. “Crossover designs are also twice as long for each subject and thus can be more burdensome for the participants. The advantage of parallel group designs is that there is no need to worry about carryover effects, although they tend to require more subjects than crossovers to obtain the same power.”
Whichever approach the investigator chooses, there are steps they can take to improve the odds of returning accurate results. As with any trial, they can maximise the internal validity of the study by using randomisation and blinding techniques. For pain trials in particular, it’s important to train participants on how to fill in the pain questionnaires, promoting a more consistent rating system for each subject.
With POC studies, the aim is to make a ‘go/no-go’ decision (ascertaining whether or not to proceed to the next phase). This being the case, you need ground rules in place from the outset, laying out what sort of results you would require in order to move onwards. Typically, a treatment effect is classed as significant if the p-value is less than 0.05 (essentially, a measure of whether the findings could possibly have occurred by chance). But, given the conditions specific to POC trials, investigators may wish to vary that threshold.
“For example, in a condition where there are very few treatment options, it may be more desirable to have a false positive than a false negative result at the POC stage,” says Gewandter. “In this case, investigators may want to set the significance threshold at 0.10, and loosen the restrictions on the false positive rate. These rules should be specified in advance to increase the validity of the conclusions.”
Similarly, you can limit false negative conclusions by including an active comparator or positive control – a treatment that is known to work for a given condition. If the study shows no difference between the positive control and the placebo, then it can be concluded that a negative result for the candidate drug is also a false negative.
One for all
Several new design approaches are also beginning to gain traction. One of these is the enriched randomised withdrawal design, which addresses the issue – endemic to pain trials – of treatments working only for a subset of patients.
This technique is not without its pitfalls: the trial results are less easily generalised to an entire population with a given condition, and it may not provide a true indication of any adverse effects from a treatment. Nonetheless, this design could increase the chance of spotting results in a randomised sample.
Another is the sequential parallel comparison design, which has been used in psychiatry trials and holds promise for analgesia too. Designed to minimise the placebo effect, it can theoretically reduce the number of participants, and ensure the study population is less responsive to the placebo.
“In this design, participants are randomised in the first phase to active treatment or placebo, and those in the placebo group in the first phase are re-randomised in the second phase,” explains Gewandter. “Second phase data from only those who are ‘placebo non-responders’ in the first phase is included in the analysis. This could lead to a decrease in the overall placebo response of the trial and thus increase the assay sensitivity or ability to see a difference between treatment groups.”
It should be clear there is no such thing as a perfect POC design – with so little information at their disposal, investigators cannot expect to obtain infallible results. However, by investing wisely in this part of the process, and identifying the most efficient and powerful designs for their purposes, they stand to reap rewards later on. A strong methodology should enable them reduce late-stage attrition while increasing their odds of spotting genuinely efficacious treatments.